I’ve always been a slow adopter. Uggs? Never had them. The curly plastic hair tie? I was gifted one once. I donated it. Pickleball? Haven’t played a single game.
So every time I hear that I should be using AI or ChatGPT at work, it’s not that I roll my eyes… but late adoption of trends is how I’ve generally lived my life. For things like using AI in assessment, I want to understand and master before using, but on the shoulders of people who have demonstrated that it’s worth my time to do so.
When I heard the staff member in the office across the hall from me was doing a presentation on how to choose the right AI tool for your assessment - I thought hey, here’s someone who sounds like he’s done some work. I can easily spend an hour, find a new building on this campus, and learn something that might be applicable while deepening a relationship that might be important when it comes time for our next accreditation visit.
The presenters were Joshua Wilson, Associate Professor in the College of Education and Human Development and Kevin R. Guidry, Associate Director of Educational Assessment in the Center for Teaching & Assessment of Learning, both at the University of Delaware. Talk about an hour well spent. I appreciated their framing and structure so much I thought you would as well. They were primarily focused on a faculty audience, so I’m sharing their framework with connections and applications to our work. A link to the full presentation can be found at the end of this post.
The presenters used an assessment design framework (Bearman et al., 2014) based in Australian academic assessment literature to structure their recommendations. The framework discusses six categories of decision making around assessment design: Purpose of assessment, context of assessment, learner outcomes, tasks, feedback processes, and interactions. The presentation I attended adapted these considerations and added an additional category. Below are the categories of the framework they argued as being most strongly affected by AI highlighted in bold and italics:
-
Assessment Purpose
-
Pedagogical Alignment
-
Technical Robustness
-
Ethical Considerations
-
Explainability
-
Community & Stakeholder Engagement
-
Evaluation & Continuous Improvement
I’m going to limit what I share to those things most applicable to the student affairs context, but I would encourage you to watch their full presentation.
One note before jumping in - both the talk and this blog post really focus on considerations when it comes to data analysis. As outlined in a recent Structured Conversation, there are many other ways that you can use AI across the assessment cycle.
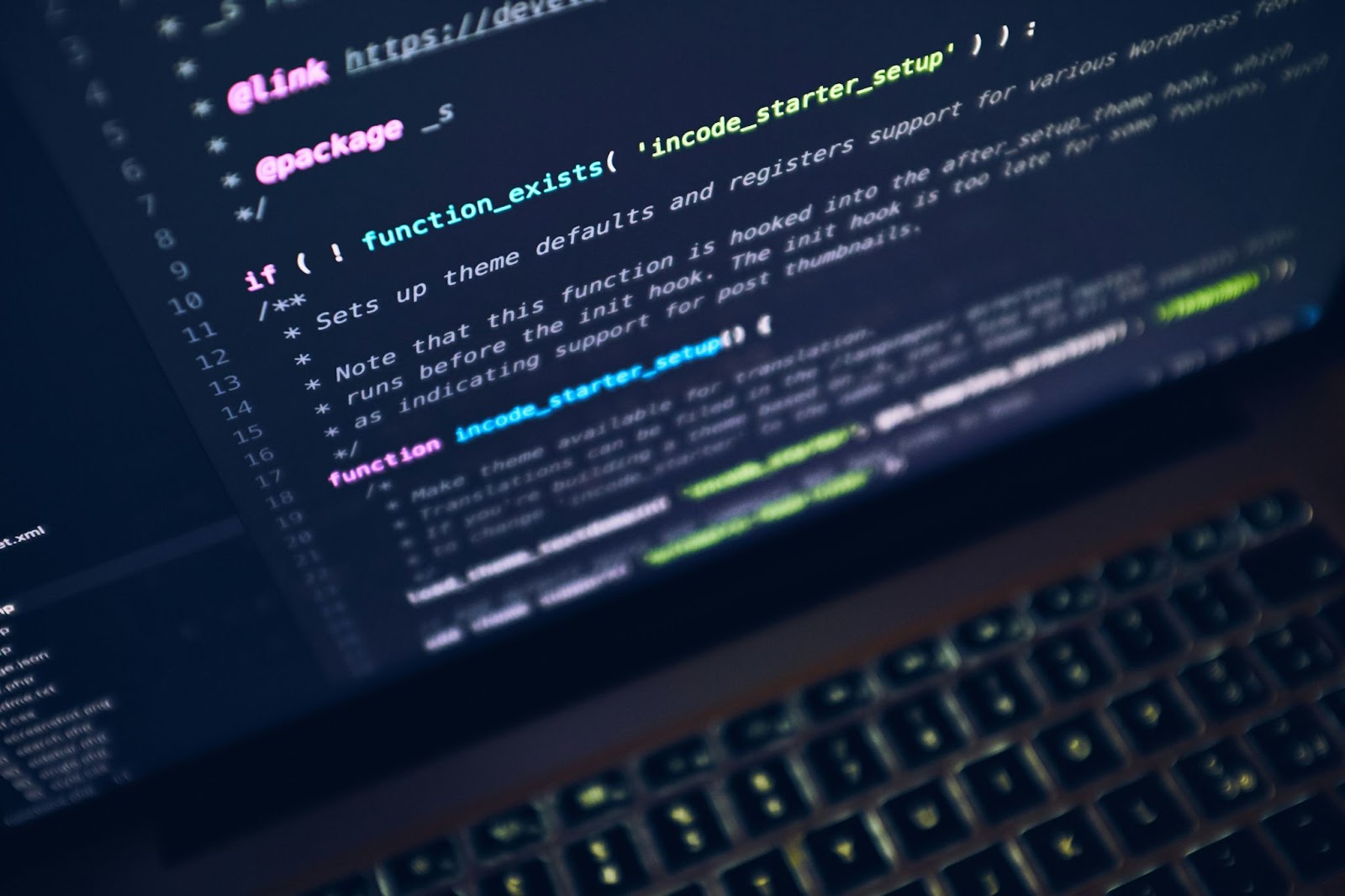
Technical Robustness
Big Idea: Consider the technical aspects of the tool, such as accuracy and integration, when deciding whether to use AI in assessment (Wilson & Guidry, 2023). The presenters offer three things to consider when thinking about the technical robustness of a tool: reliability, relevance, and the original intent of the platform. In the student affairs context, reliability is the most applicable of these. They also pointed out that a tool that integrates well with your campus LMS is probably more feasible to use within the academic assessment space.
One thing you’ll hear often is that those doing student affairs assessment can throw some open-ended responses into a generative AI tool (e.g., ChatGPT) and have it do the analysis for you. One key challenge that won’t be new to any qualitative researcher is the desire for content or performance to be treated or graded similarly regardless of who is looking at the content (reliability). When using a team of staff to evaluate portfolios or interview transcripts, we hold score calibration meetings. We typically provide a training data set and discuss our process to ensure those who will be evaluating the information will evaluate it similarly. If we're working in a content analysis framework we'll also calculate interrater reliability and perhaps revise our codebook to ensure we reach an acceptable interrater reliability. The presenters argued the same process is needed when using AI. If I ask an AI tool to evaluate an open-ended response and let me know if the author understands the key components of social justice and my Analyst uses the same tool with the same data to ask the same question - we should get the same result. And if we did that 100 times, it should be the same every time. You shouldn’t just upload qualitative data into ChatGPT, ask it to code it, and then take the output and move on to your report. You need to ensure that the technical process behind the scenes (on any platform you might use) is going to give you consistent results. If possible, you may need to spend time teaching the AI tool how to evaluate the content you provide appropriately, and in turn, you need to evaluate the tools ability to do so before you accept the results. You may get out of the headache of scheduling those coding calibration meetings, but you can’t skip this step in the process. Additionally, doing this type of comparison may not even be possible with some tools which makes their use questionable.
The speakers also highlighted that using an AI tool already built into your campus LMS facilitates an instructor’s use of that particular platform to grade assignments. I wanted to highlight this because having an integrated tool also means that your institution has evaluated and approved that tool for use with assurances that the company that maintains that tool will respect the confidentiality of your data. Your Information Technology, General Counsel, and Procurement teams have decided that the platform is appropriate, secure, and worthwhile to campus operations. This point overlaps nicely with the next category, ethical considerations.
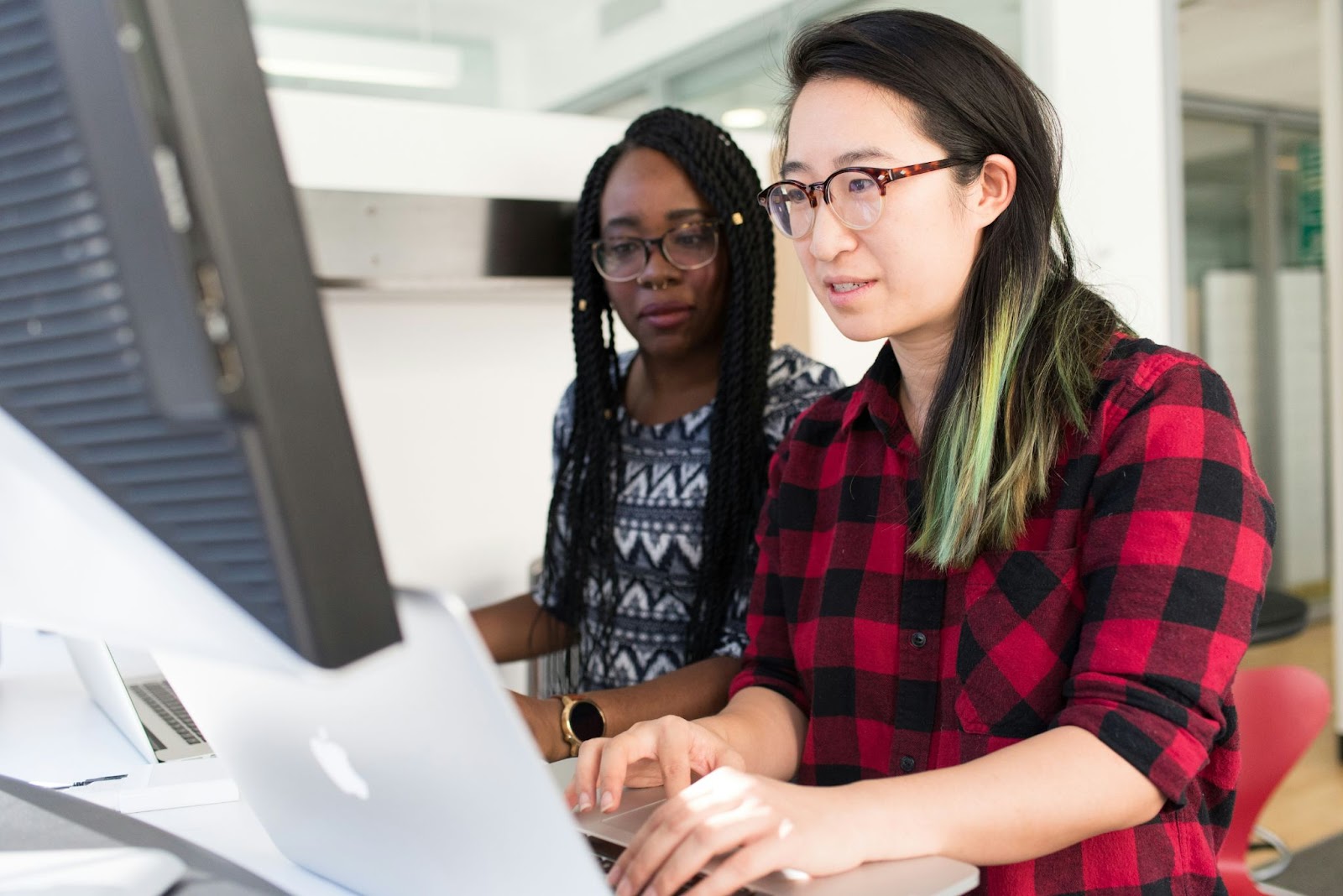
Ethical Considerations
“Big idea: Equity, access, and data privacy must be carefully considered for automated/AI assessments” (Wilson & Guidry, 2023). In every conversation about AI, at some point the ethics questions come up. In conversations around AI in student affairs assessment, this is one space in which I worry we don’t spend enough time.
Similar to above, when using an AI or other automated tool for assessment purposes, we want to make sure the output is equitable and bias is minimized. The tools we use are only as good as the humans who build them, and the idea of
algorithmic bias has been written about extensively. When considering giving an AI tool qualitative responses, even in the cases where we are being intentional and ensuring the AI tool is calibrating scores appropriately based on past data we’ve collected, how are we accounting for student demographic differences and approaches to answering a question? How is training an AI tool using past data not validating the work of the majority? What systems and processes are we building in to check the output?
If you are going to be putting student data into an AI tool, you need to be aware of how the tool will view that data, what it does with the data, and other privacy considerations. In some cases, the company that built the tool now owns that data. This again is where approval of various campus entities like General Counsel and Information Technology should be explored; some institutions are even creating Chief Privacy Officer roles that you may be able to consult. Considering the type and level of assessment is also crucial as this may impact those of us doing assessment at a divisional level or those within certain functional areas more than others. There may be times where using AI to code qualitative data is relatively ok: maybe you asked a group of student leaders three things they learned as a result of attending a conference and you want the tool to group those responses. You’ve read them already and know that the responses are things like “communication” and “leadership transition practices for my organization.” On the other hand, I’ve read open-ended comments associated with an RA evaluation that had us on the phone to our conduct office and Title IX staff. Even though putting all the responses into a tool for faster thematizing would have saved me a lot of time in making suggestions about topics for the next RA training, I may have missed those critical details, not to mention if the students’ making the Title IX claim had referred to the alleged assailant by name. Although it may make our lives easier, even deidentified data entered into a 3rd party platform is often not good practice.
Mostly I hear about issues of access from the student perspective - if we are asking students to complete projects using AI tools but there are access inequities (e.g., technology access), this will result in inequitable outcomes. However, I also wonder about equitable access for staff. Some platforms like ChatGPT have a paid version with significantly more features. Although some campuses have allowed staff to use campus resources to pay for tools like ChatGPT and
others have entered into enterprise license agreements providing this resource to all employees, access may still be restricted or unclear at many institutions. If I want access to it but it is not a university approved tool, I would need to pay for that out of pocket. How does my positionality and financial privilege then influence what I am able to accomplish in my role versus someone who doesn’t have the personal funds to cover this cost? How will this impact how productive I am perceived to be compared to my less financially privileged peers and what implications will that have on merit raises or promotions? As the technology changes quickly, different institutions will necessarily have different access.
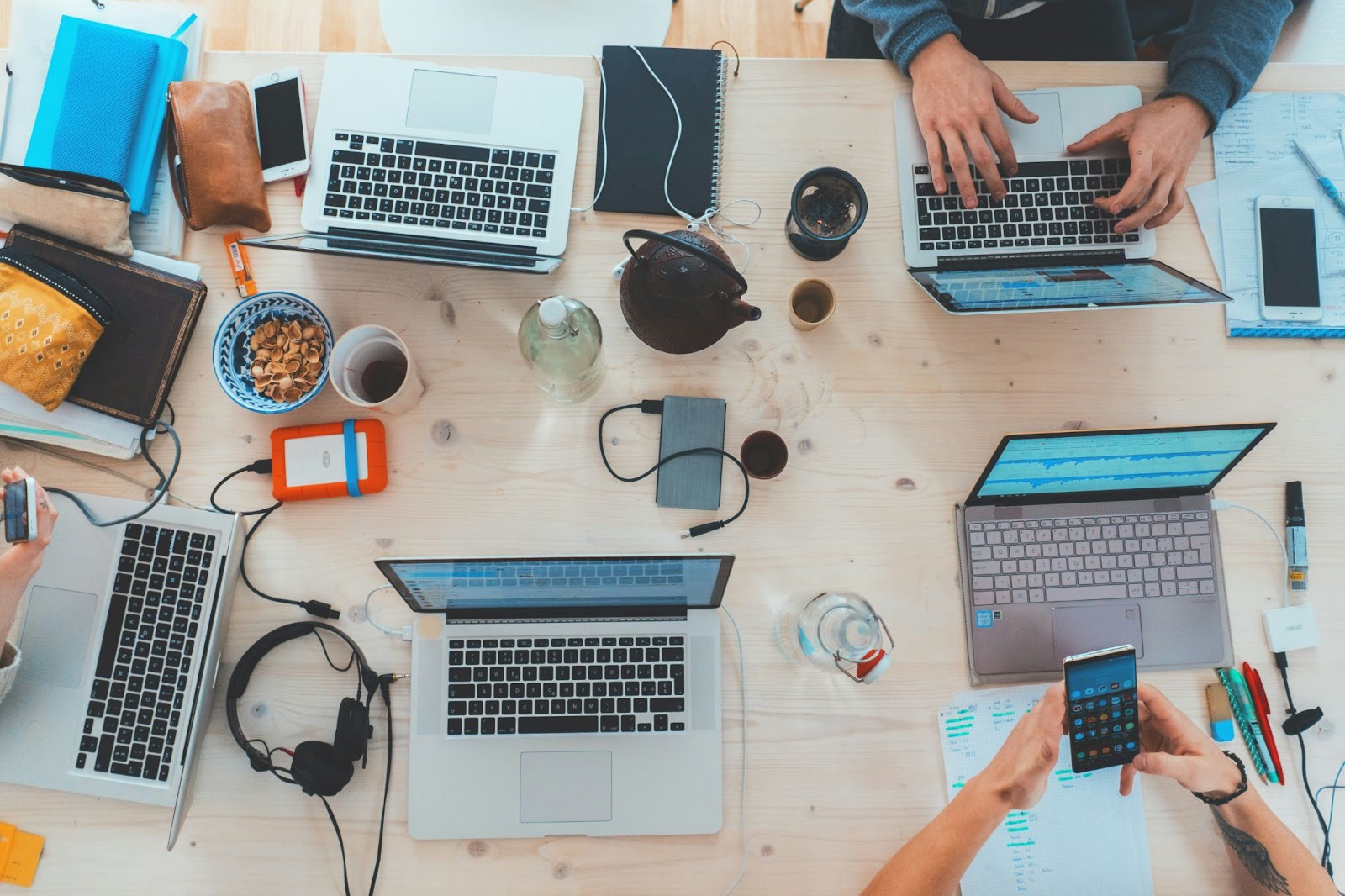
Community & Stakeholder Input
“Big Idea: Students, as those most directly affected by the automated/AI assessment should have opportunities to share their perspective and feedback. Their feedback should be carefully weighed” (Wilson & Guidry, 2023).
This is the other space where equity-centered assessment and AI intersect. Often in our equity-centered conversations we talk about including students in the assessment design process but this is rarely mentioned when we start talking about AI specifically. How are we getting feedback from students and our colleagues across the institution about comfort with using AI tools to do our work? Are we including consent statements on surveys that let students know we will be putting their responses into an AI tool? As Gavin pointed out in a recent
Structured Conversation, even if we provide this consent language, do students know what that means and can we really consider this to be informed consent without doing a bit more education? What does it mean to have community and stakeholder input in our assessment design when some of these tools are so new and changing so rapidly that it takes a lot of time to stay on top of these changes? I sometimes wonder - if I adopted an AI tool for analysis, will this free up some of my time… only so that I can spend time learning, thinking, and talking about AI?
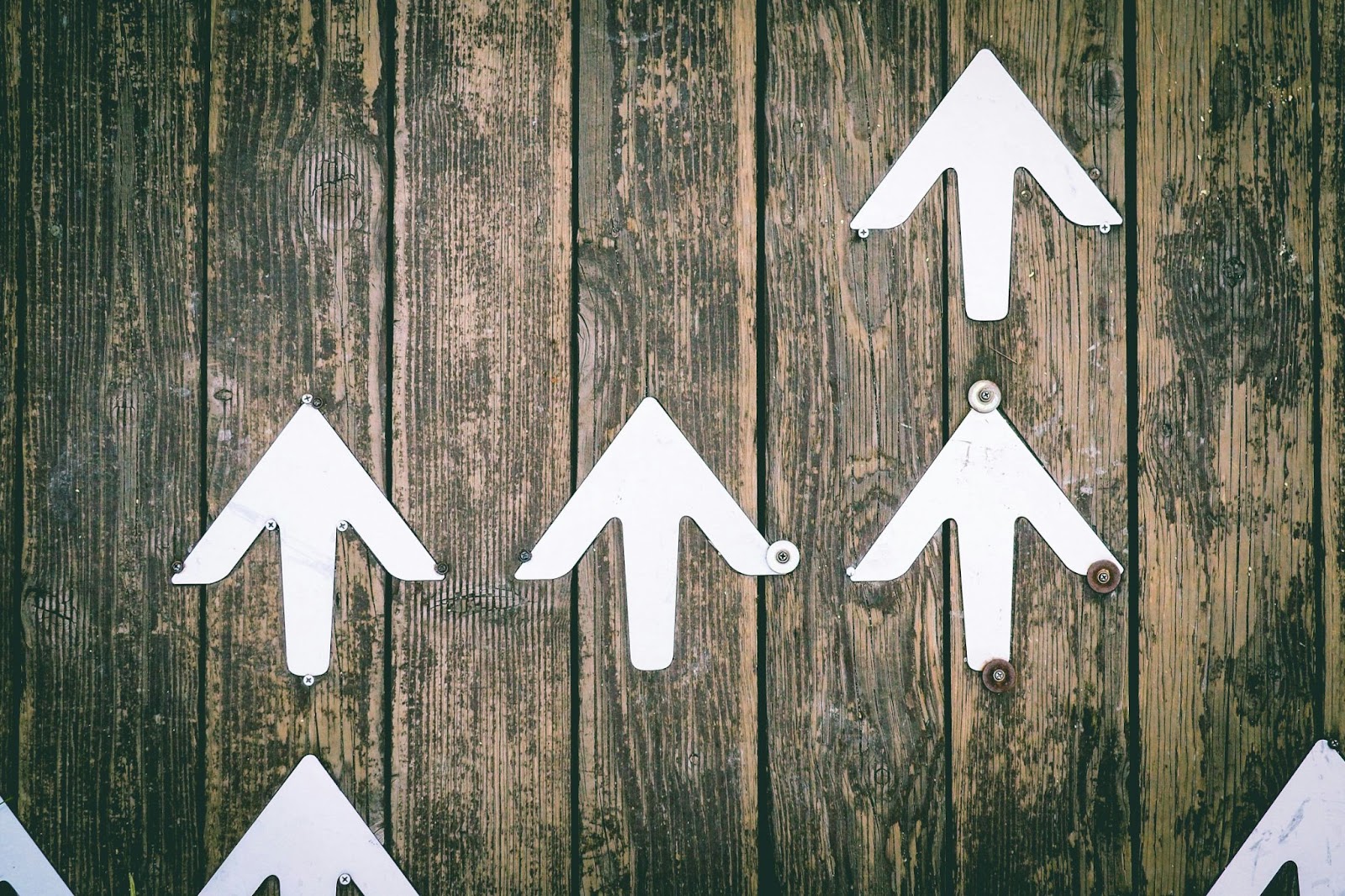
Evaluation & Continuous Improvement
“Big Idea: Pilot → evaluate → adjust and improve → rinse and repeat” (Wilson & Guidry, 2023).
This should be nothing new to this group, but of course needs to be mentioned. These tools are evolving so rapidly and our adoption within student affairs assessment is so new, we will need to do what we do best and evaluate our use of these tools. As with everything, having a group of students, peers, and colleagues reviewing our uses of AI, monitoring if it is actually saving us time or just shifting how time is spent, and making a determination on how and for what types of projects you will use AI tools for is critical.
I’ll also add that for those of us in divisional or supervisory roles, we probably have some responsibility to make sure staff are using these tools appropriately, ethically, and in line with how our institutions believe they should be used. We might have staff who engage with SAAL or want to move into assessment roles who are excited by the possibilities AI provides but aren’t able to spend the time engaging as deeply with the ethical and legal implications of AI as we might be. Monitoring and evaluating the use of AI in assessment across the division might be something you consider.
How I’m using AI in my own work:
Taking all of this and what else I’ve learned about AI in student affairs assessment, it’s natural to wonder how I’m using it in my work. Personally, I do not feel comfortable putting any data, quantitative or qualitative, into an AI tool right now. The University of Maryland released a
statement last May that is short and clear - they are advising members of their campus community to not post anything into a generative AI tool that is not appropriate to post to a public website. Most if not all raw data I would collect in my role, even de-identified, I would not be willing to post to a public website, so I continue to believe that this is the right decision.
In my recent post where I talked with Shaun Boren about how his team is using AI, I appreciated his example of giving only variable names and asking a tool like ChatGPT to develop the syntax and troubleshoot errors. I will probably explore that use case in the near future and I have used it here and there for things like a presentation outline or rubric creation, but I’m still exploring the possibilities. I don’t doubt that I will use AI more and more in my work, but I want to better understand and feel more comfortable with the potential ramifications before I do.
Interested in learning more? I really encourage you to
watch the whole talk (37 minutes) for additional considerations.
Many thanks to Kevin Guidry for feedback and notes on an early version of this post
Sophie Tullier, SAAL Blog Writer and Director of Assessment, Data Analytics, & Research at the University of Delaware